Time to read: 7 min
Over the last few years, there’s been significant progress in the fields of robotics and artificial intelligence. While Robotics-as-a-Service (RaaS) has boomed in the past decade thanks to fresh financing models, broader adoption of industrial robots remains elusive. While demand exists, many RaaS providers have found it challenging to deploy robotics effectively for real-world tasks — ones that humans have been performing for a long time.
The Limitations of Rigid Robots
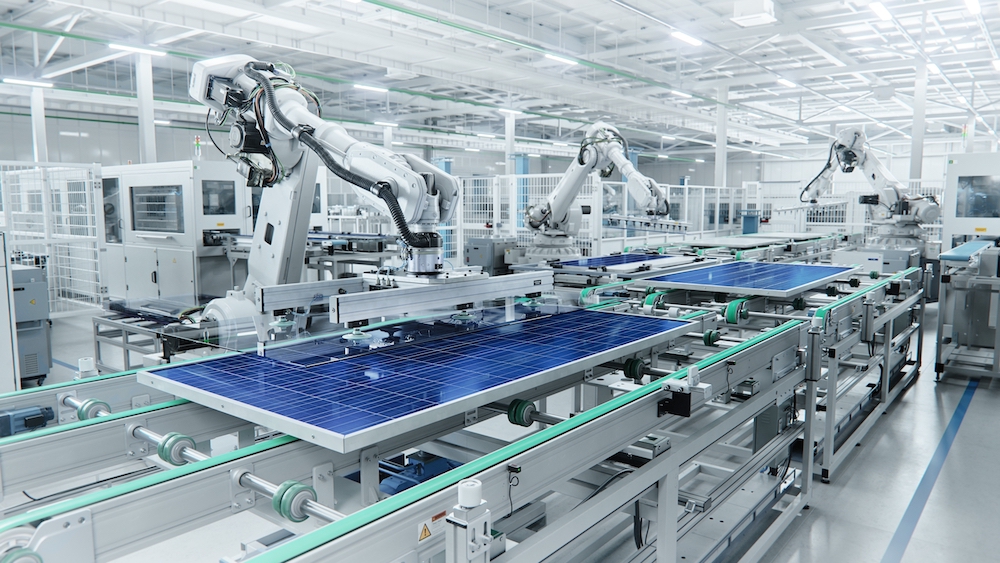
Wide Shot of Solar Panel Production Line with Robot Arms at Modern Bright Factory. Solar Panels are being Assembled on Conveyor.
Robots, as most know them, are rigid and high-strength, capable of repeatedly performing tasks with precision and accuracy. However, this rigidity has its drawbacks when robots encounter unexpected conditions, like collisions with external objects (or people). In exchange for enhanced force and positional control across every degree of freedom, rigid systems require complex machinery and expensive, reliable, and high-frequency control loops to ensure proper operation and prevent accidents.
We’re proponents of practical robotics technologies, and are intimately familiar with these trade-offs.
Having published dozens of patents and written papers on complex and expensive robotic force-feedback systems, it’s clear that such systems take months or years to perfect, but then can repeat tasks, nearly flawlessly, for thousands or millions of components. That’s great if you’re Tesla, Samsung, or Apple and the upfront NRE (non-recurring engineering) costs can be amortized over many identical components. However, it’s not so great if you’re producing at lower volumes or a higher mix, like most other manufacturers.
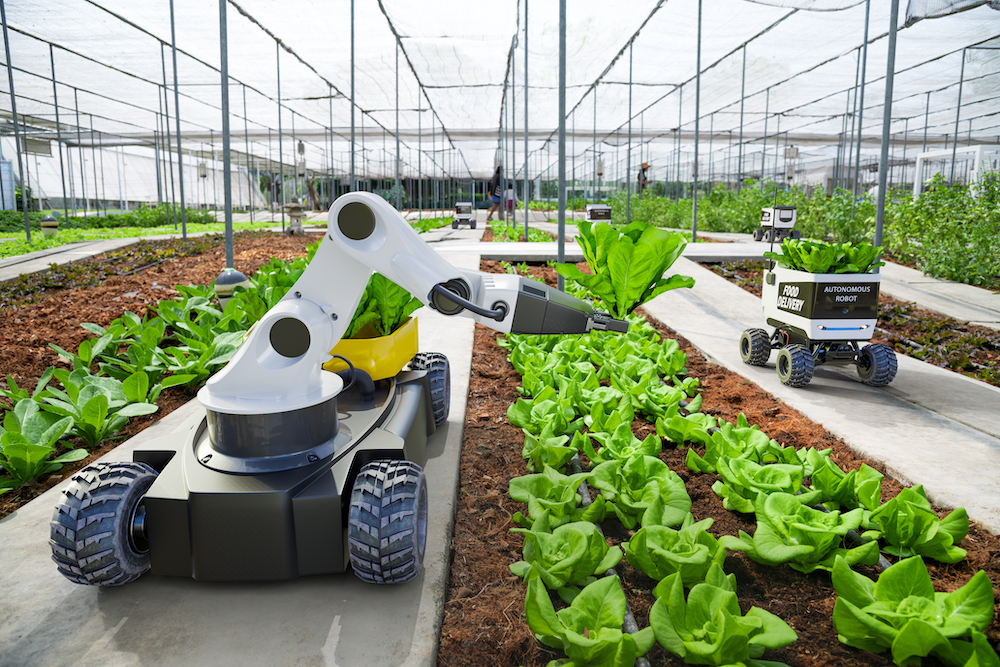
Of course, human operators take a few hours or days to train, even as they’re less reliable and precise than their robotic counterparts. That short training and set-up time is why industries such as manufacturing, construction, agriculture, and food processing have relied on human labor for so long, despite the potentially huge addressable market and the dirty, dull, and dangerous nature of a lot of this work.
The intricate control infrastructure requirement makes the application of conventional, rigid robots to real-world tasks both daunting and resource-intensive, and has limited their broad adoption across industries.
Moravec’s Paradox has long stood as the central tenet explaining why it’s so difficult to train robots. It suggests that reasoning tasks we humans find complex, like strategic decision-making or chess-playing, are relatively easy for AI. Yet seemingly simple tasks for a human, like recognizing a face in a crowd or navigating a cluttered room, are especially challenging for a machine. This paradox results from our evolution — millions of years have fine-tuned our sensorimotor abilities, while our capacity for high-level reasoning is a far more recent development.
One of the major reasons for these challenges is that humans have so many sensory inputs that are hard to mimic in robotics. We vastly under-appreciate just how many sensory inputs a human receives when completing any sort of task, and these inputs are incredibly difficult to replicate — both individually and as a whole — in robotics. As a result, it’s challenging for robots to carry out human-level, fine-tuned sensorimotor abilities at scale in a generalizable way.
For many years, the implications of Moravec’s paradox have constrained the capabilities of rigid robots and confined them to carefully controlled environments and specific, repetitive tasks. However, the rise of AI and reinforcement learning has provided us with a unique opportunity.
AI and Reinforcement Learning Unlock Soft Robotics
AI is still in its infancy, yet a wave of much more accessible AI models is now available. And with innovations in advanced materials and soft actuators, a future with AI-powered, soft robots aware of their surroundings will revolutionize industries and redefine human-robot tasks.
Unlike their rigid counterparts, soft robots are crafted from compliant materials that replicate the flexibility and adaptability of living organisms. A shift towards softer, more human-like robots opens a way to leverage reinforcement learning for training, path planning, and optimization.
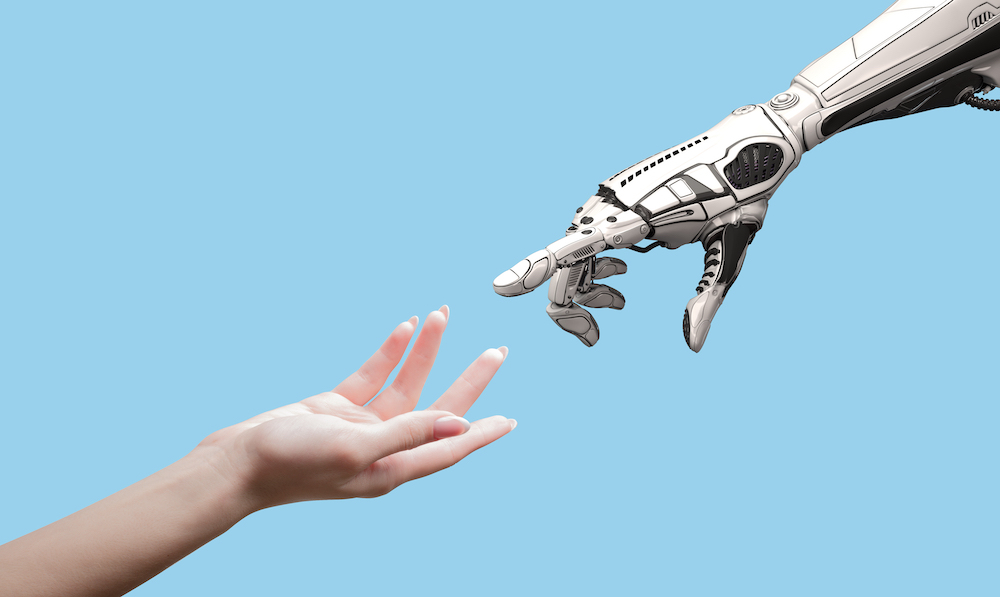
Just as children learn through observation and practice, malleable robots enhance their skills and understanding by observing human actions and learning from trial and error. This capacity for continuous learning and adaptation reduces the risks associated with robot training collisions — it turns potential disasters into mere bumps in the road.
The combination of soft robotics, advanced machine learning techniques, and increasingly abundant computational power allows us to develop robots that can learn and adapt in real time, mirroring the way children learn to interact with the world. Through observing humans, they can grasp the nuances of natural movement, navigate complex environments, and even anticipate and respond to changes.
That ability to interact safely and seamlessly within existing human-centric environments is a huge advantage — soft robots can navigate spaces and interact with people effortlessly, thanks to their compliance and adaptability.
And soft robotics can work well in conjunction with rigid robotics, too, whether as an end effector or a more deeply integrated component of the system. Combining soft and rigid robotics leverages the strengths of both — interacting components (whether interacting with a human or a delicate object) can be soft and compliant while maintaining the strength, precision, and accuracy of a rigid system.
Solving the Door Handle Problem
Opening a door handle is a common task that requires a delicate balance of force, precision, and adaptability — yet even the world’s most advanced robots still struggle to open different kinds of physical doors. That’s why it’s a perfect example to use when comparing the performance of a human-like, soft robot to a rigid robot equipped with force feedback.
How Rigid Robots with Force Feedback Open Doors:
A rigid robot with force feedback relies on its precise and accurate movements to interact with a door handle. This type of robot typically uses a predefined set of instructions to approach the handle, then applies force to grasp and turn it. The force feedback mechanism allows the robot to sense the amount of force it is applying and adjust accordingly to avoid damaging the handle or the door.
However, if the robot encounters an unexpected situation, such as an unusual door handle design or an obstacle in its path, it may struggle to adapt its approach and fail to complete the task.
How Human-Like Soft Robots Open Doors:
In contrast, imagine a human-like soft robot, built with compliant materials, that’s more adaptable and flexible. This soft robot uses its vision and reinforcement learning capabilities to observe humans opening doors and learn the appropriate technique — so the robot can better understand various handle designs and adapt its movements accordingly.
When approaching the door handle, the soft robot’s compliant nature allows it to conform to the shape of the handle and maintain a secure grip, regardless of the handle’s design. Since the robot is similar to humans in terms of stiffness, it can apply force to the handle in a more nuanced and controlled manner than a rigid robot can. Also, its reinforcement learning capabilities also allow the soft robot to adapt and optimize its actions in real-time based on the specific situation.
While a rigid robot with force feedback can execute the task of opening a door handle with precision and accuracy in a perfectly-known and repeatable environment, it’s likely to struggle in unexpected situations.
On the other hand, a human-like soft robot is not only capable of completing the door-opening task, but is also adaptable, flexible, and interacts more intuitively with its environment — even if that environment changes (as all human-centric environments tend to).
Plus, soft robotics are often pneumatically actuated to grasp objects, which allows for the easy addition of suction capabilities. This combination of grasping and suction, along with a closed loop system powered by sensors and AI, makes for a truly flexible robot that can easily grasp a wide range of objects — everything from an orange or an iPhone, to a delicate wine glass or a door handle.
Shifting the Focus from Precision to Adaptability
As developments in reinforcement learning, computer vision, sensors, and soft robotics progress, we expect the practical and commercial applications of soft robotics will be an order of magnitude greater than those of rigid robotics. These will supplement, but also challenge rigid robotic systems.
By shifting the focus from precision to adaptability, soft robotics aided by AI can turn Moravec’s paradox on its head and enable robots to learn and perform a broader range of tasks in real-world environments — it holds tremendous potential for the future of robots and our interactions with them.
As builders and investors, we’ve seen the field of soft robotics develop significantly over the past decade. But commercialization of the technology has been sluggish relative to the significant research developments driving the field forward, and the number of new soft robotic startups is small relative to other robotics and alternative robotics companies.
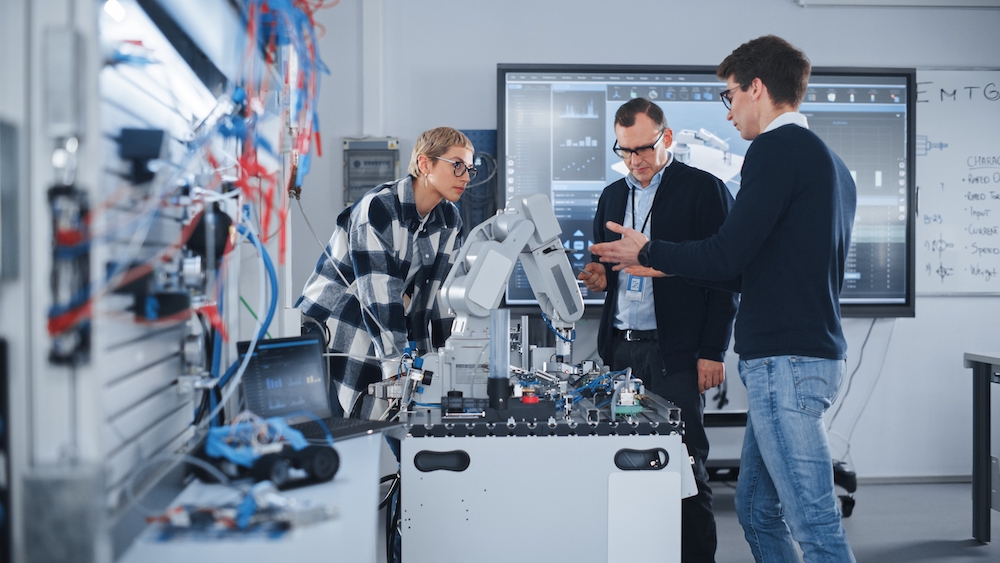
But the kickstart required for widespread commercialization of soft robotics might not even be the developments within the field. With recent advancements in AI, computer vision, and reinforcement learning, soft robotics can now combine those technologies to create proper closed loop systems and unlock the true potential promised by soft robotics in the first place: fully conforming and flexible soft robotics that deliver on the promise of automation in human-centric environments at scale.
It’s time we embraced the future where these technologies will redefine the very essence of a “smart” robot. Adaptability is paramount in this brave new world where we expect to see many interesting soft robotic startups in the coming years — and we’re excited to help those founders build the future of robotics.